Causal inference in computational and systems biology
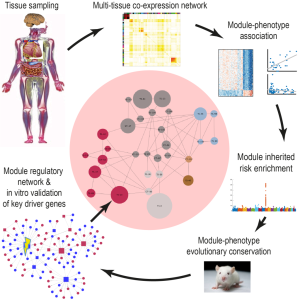
Our group has a broad range of research interests in bioinformatics, computational biology and machine learning. In biology, our main interest is to understand gene regulation and how it is affected by genetic and epigenetic variation.
In other words, how does the genome determine which genes are expressed (active) in different cell types, and how do genetic differences between individuals lead to differences in gene expression and ultimately to differences in health and disease traits?
We use machine learning approaches and large sets of genetic, epigenetic, and other molecular data to answer these questions. Machine learning is a field at the interface of computer science and statistics that aims to identify correlations and other meaningful patterns in large data sets. Biology is an ideal area for testing and developing new machine learning algorithms, because in biology correlations alone are never enough. For instance, to know that high cholesterol and high blood pressure are often seen together in people with diabetes or heart disease is not very useful, until we establish that in fact, high cholesterol causes high blood pressure, and should therefore be the therapeutic target. To establish similar causal relations at the level of genes, where thousands of genes are expressed in every cell of our body, influencing each other in untold ways through complex, unknown networks of genetic interactions is the challenge that we aim to address.
In short, to paraphrase a well-known saying: nothing in biology makes sense, except in the light of causal inference.